Artificial intelligence facilitates mineral prospectivity mapping
Methods that belong to branch of computer science called “artificial intelligence” allow the production of better and more reliable prognoses of mineral deposits.
“Artificial intelligence helps process and analyse large quantities of data. When the initial data is complex and many different sets of materials are in use, methods based on artificial intelligence can be used to produce simple forecasts of where mineral prospecting should be targeted,” says Vesa Nykänen, Research Professor of Geoinformatics at GTK, the Geological Survey of Finland.
According to Nykänen, the new methods utilising artificial intelligence, or machine learning more specifically, are cost effective, speed up the process of mineral prospectivity mapping and provide reliable prognoses.
“These modern techniques allow the analysis of data in multi-dimensional feature space, which is difficult for the human brain to visualise. The computer can easily manage such problematic scenarios. It is also likely that artificial intelligence finds unexpected correlations, non-linear solutions which would otherwise remain undetected.
Open source code
GTK has been studying machine learning and its use as an aide to mineral prospectivity mapping from the beginning of the 2000s. For the past five years, researchers have concentrated on developing the required IT tools.
“The algorithms are developing rapidly. New solutions and application methods are being found all the time.” Nykänen says.
The application development work has been speeded up by the increased computing power of computers, making it possible to process large amounts of data efficiently.
The researchers at GTK have developed tools for geologists, available as open source code in the GitHub network. Anyone around the world can download the code and participate in its development.
The code development work was initially started by researchers in the United States Geological Survey and the Geological Survey of Canada in the late 1990s. From 2005, the code was maintained and developed by the University of Campinas in Brazil.
GTK has been maintaining the code and developing new tools for it since 2016.
Aided by machine learning
Machine learning is increasingly being used in algorithm development work. Depending on the data used, the algorithms can be either guided or unguided.
If there are known mineral deposits in the area of interest, they can be used for teaching and guiding the algorithm. The model learns from existing data and is capable of producing, on that basis, a prognosis of the most promising locations where mineral exploration is worthwhile.
The alternative is to convert the skills and knowledge of the expert into a mathematical model. One example of this is the Mineral Prospectivity Modeller (MPM) Online Tool developed by the GTK for making an assessment of regional mineral potential in Finland.
The MPM Online Tool uses the geographic information system (GIS), geophysical, geochemical and bedrock maps produced by GTK and other maps based on these. The tool combines different maps by using fuzzy logic.
The tool is available at http://gtkdata.gtk.fi/mpm/ and can be freely used online. It runs on most common browsers. No additional software is needed to use the tool.
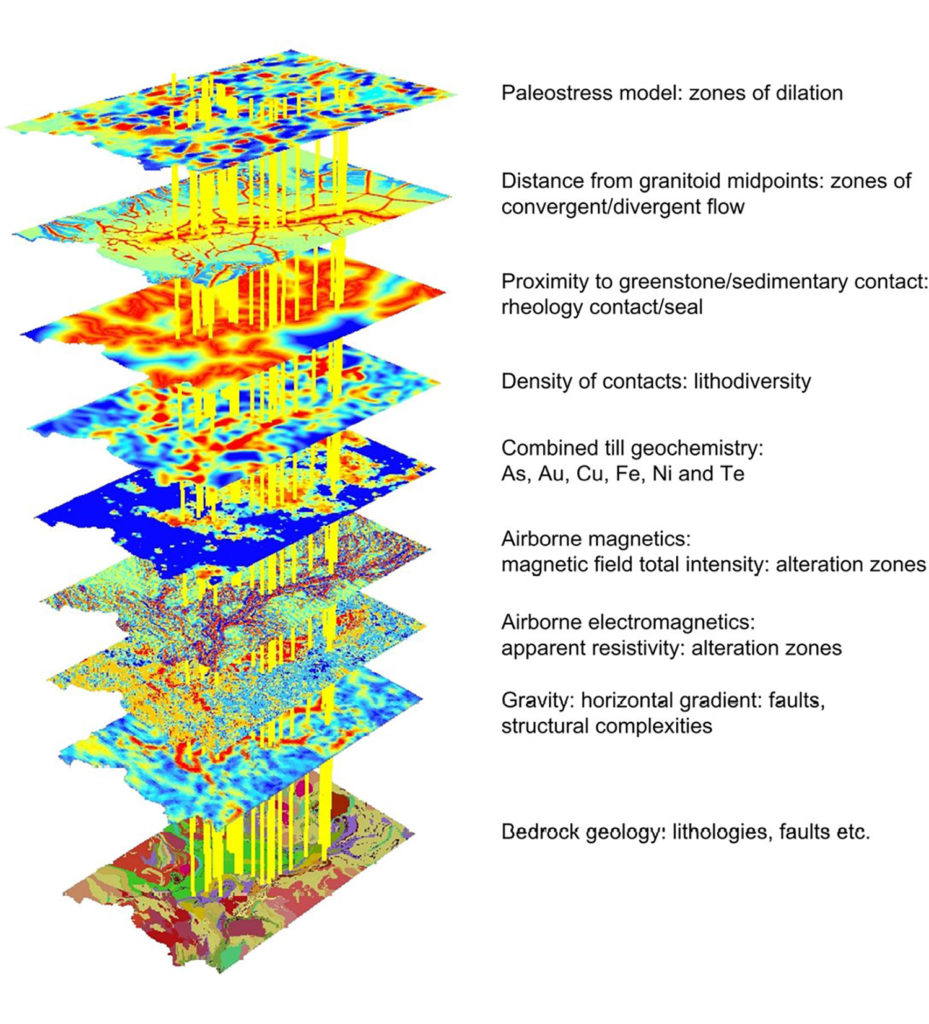
The data must be pre-processed
Whichever tool is chosen, the data is rarely used as it is.
“The diversity and quality of data, as well as its pre-processing decide how well the modelling works in the forecasting of mineral potential. Among other things, pre-processing classifies, re-scales, filters and interpolates the data,” Nykänen says.
But whether a supervised or un-supervised algorithm based on machine learning or an algorithm based on expertise is applied in the model has, at least so far, not been found to affect the reliability of the result. According to Nykänen, the results are, on average, comparable.
“It’s the data that decides the outcome. That is why one should invest resources in collecting and processing the data. Artificial intelligence does not in any means replace the experts; instead, it highlights their role. The experts collect the data and produce the maps that artificial intelligence then analyses,” Nykänen says.
In the traditional scenario, the expert would place the maps on top of each other on a light table and try to visualise the best places for mineral exploration. This step can now be completed with spatial data software and algorithms by utilising machine learning. The new systems also have the advantage of repeatability. Modelling can be later repeated, and the parameters can be changed, if required.

Multiple scales
Artificial intelligence is already used for mineral exploration in many places around the world. Consulting companies, for example, provide customers with prognoses produced by using new digital systems.
GTK has previously published prospectivity assessments for gold deposits in Central Lapland. After establishing the locations of most favourable target areas, field samples have been collected, and they have shown that the model works well: the gold content of samples has been higher than on average in the area.
GTK has applied methods based on algorithms and computer calculations for first-stage mineral exploration, i.e. used them as an aide for selecting target areas. However, the methods are also suitable for assessing prospectivity in a closer scale. The initial data can e.g. consist of 3D spatial data produced of the target.
“In addition to our own studies, we have produced prognoses for our customers by using their data. Mapping processes are currently in progress in Finland and abroad,” Nykänen says.
Over the years, the research and development projects of GTK have in particular been funded by Tekes, the Finnish Funding Agency for Technology and Innovation, whose provision of services is now being continued by Business Finland.
GTK is currently coordinating the New Exploration Technologies (NEXT) project, where new mineral exploration methods are being developed. Approximately one-third of the total funding of EUR 6.9 million is allocated to developing the analysis and integration of data. The NEXT project will be implemented with the support of the EU’s Horizon 2020 programme that funds research and innovation.
In the on-going Exploration Lapland 3D project and the Hattu 3D project implemented in Eastern Finland, GTK is developing the computational collective interpretations of large amounts of data collected from deep drilling samples and geophysical surveys. The projects apply the latest digital measurement and artificial intelligence technologies and combine the ground property data collected from the field with 3D modelling.
The purpose of the projects is to speed up and improve the accuracy of the initial stage of gold exploration in areas where little exploration has taken place earlier and where not much data are available.
The Exploration Lapland 3D and Hattu 3D projects are mainly funded by Business Finland. Several mining and mineral exploration companies are also participating in the projects and their funding. The projects are also supported by the European Regional Development Fund.
Invitation for the scientific community
The amount of available data is likely to rapidly increase in the future. At the same time, new modern mineral exploration methods and sensors will be introduced. For example, data produced by satellites can be used in areas where the bedrock is not covered by topsoil and vegetation.
The increasing amount of data will expedite the need to develop analysis methods based on artificial intelligence and machine learning. Nykänen would like to see the entire international scientific community participating in the development work.
“The development work for Linux is a good example of the power of crowdsourcing. When code is developed in an open environment, something new and great can be achieved. Cooperation is probably the scenario that can open new avenues for mineral prospecting,” Nykänen says.
Text: Timo Hämäläinen